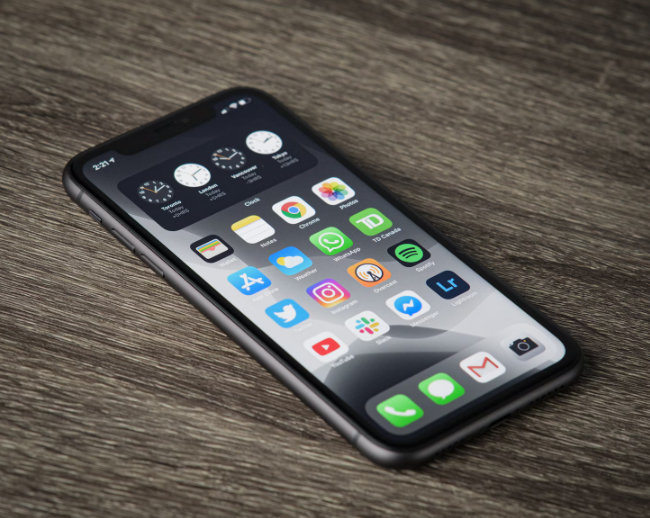
In the ever-evolving landscape of sports betting, technological advancements have propelled the industry forward, transcending geographical boundaries. Betting apps South Africa may not drastically differ from their American counterparts, yet they play a pivotal role in shaping the betting experience on basketball and other sports. In this article, we’ll delve into the realm of basketball betting apps, drawing a parallel between the user experiences of bettors in South Africa and the United States.
Continue reading “Exploring the Convenience of Basketball Betting Apps”